The state of technology has not always been like this. In fact. technology and innovation have come such a long way that we have almost forgotten the dark and unforgiving history that it holds. The rapid normalization of technology over the past few decades has left little to no room to decrypt its advancement and analyze the ethical and moral aspects of it through past historical events. While it is impressive how far we’ve come with technology, we shouldn’t forget that history repeats.
We’ve been diving headfirst into the AI market without entirely understanding the technology behind it. The market is already worth billions now, and it feels too perfect to fail. It might seem like an unprecedented breakthrough but it’s not. Something similar happened back in the 90s with the dot-com boom. Perhaps stories of it could provide a perspective on the potential consequences AI could hold.
Where It All Began
Artificial Intelligence (AI) is not a new concept. It has been around in some form or the other since the advent of computers. However, the AI we hear about today is far more advanced than the past. It’s no longer just simple bots or video game enemies; we’re talking about large language models (LLMs) and neural networks like ChatGPT, which seem to possess a level of sophistication and intelligence that was once the stuff of science fiction.
When AI first started gaining traction, it was both intriguing and mystifying. The claims were bold : AI would soon transform industries, create new job opportunities, and even help solve some of the world’s most pressing problems. Corporations were quick to latch onto these promises and they were delivered for the most part. Nvidia, for example, went from being a successful GPU producer to becoming one of the world’s largest companies, all thanks to its AI endeavors. Microsoft similarly bet big on AI early on, integrating it into every corner of its operating system, and is now bearing its fruits.
But then there’s the other side of the coin. Despite all the hype, there are plenty of voices warning that AI might not be the revolutionary force it’s made out to be.
Some argue that much like the dot-com boom of the late 1990s, we’re experiencing a bubble.. Back then, investors believed every new website would make millions, only to see the bubble burst, leaving many businesses in ruins.
The Tech Industry’s Culture
The culture of Silicon Valley is unique. It’s a place where showmanship often trumps actual innovation, and billion-dollar valuations are more a product of storytelling and media manipulation than genuine technological advancement. Individuals can become millionaires overnight, and technical ideas often overshadow sound business fundamentals.
The relationship between venture capitalists (VCs) and tech founders is symbiotic. VCs need radical ideas to invest in, while founders need capital to bring their visions to life. This dynamic often leads to the survival of unprofitable companies through continuous injections of capital, inflated valuations, and media hype, all aiming for an eventual IPO or acquisition.
The Role of Venture Capital
Many VCs come from backgrounds in Wall Street or entertainment, lacking actual tech experience. This creates a scenario where both sides—VCs and founders—are essentially “the blind leading the blind,” performing their roles in hopes of reaching a significant payday. Inspirational speeches, motivational essays, generous salaries, and flashy perks are all part of a deliberate strategy to keep a steady flow of new graduates entering the tech sector.
The tech sector’s allure is maintained through these tactics, ensuring a continuous influx of talent. However, the cycle of hyping new technologies without delivering substantial results is problematic.
Tech Hype Cycles
In tech, you only need one big win. This leads to a vast pool of aspiring founders and VCs, all eager to find the next big thing. The sector is quick to shift focus to new trends whenever the market becomes skeptical about the current ones.
In the early 2010s, big data was hailed as a revolutionary technology capable of uncovering deep insights from massive amounts of data. It promised to transform various sectors, from predicting demand to preventing crime. Similarly, Software as a Service (SaaS) was promoted as a resilient, high-margin business model.
Despite the hype, many big data and SaaS companies struggled to deliver on their promises. The majority of consumer startups and SaaS companies continued to bleed money years after their IPOs, even in favorable market conditions. This cast doubt on whether these technologies could live up to their ambitious claims.
The Rise of AI Hype
In 2022, the AI hype began with the release of ChatGPT. Suddenly, every tech company rebranded as an AI company, and having an AI strategy became essential for Fortune 500 companies. VCs shifted their focus to AI startups, and the public was convinced of AI’s potential to take over jobs causing millions to lose their source of income through the incessant fear-mongering. Silicon Valley figureheads performed in front of Congress, urging for regulation and protection for workers that will potentially be displaced by AI. This performance convinced both the public and the government of AI’s immense potential, despite lacking substantial evidence.
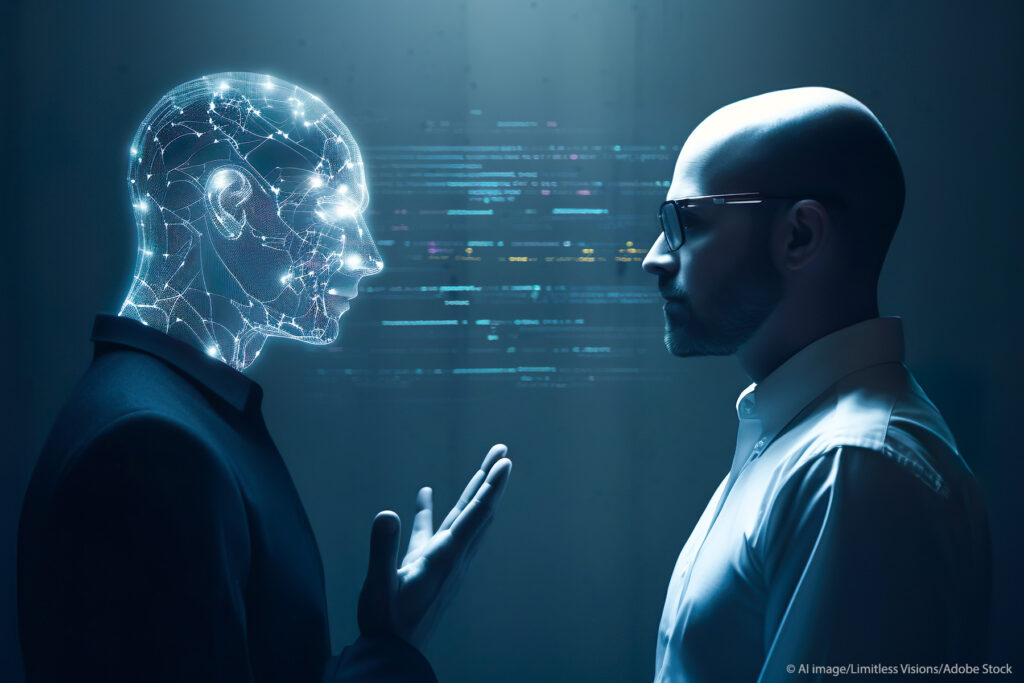
AI as the Latest Pump-and-Dump
If we think about it, AI is just the latest in a series of tech trends designed to maintain hype and high valuations and drive the endless cycle of capitalism. Before AI, there was crypto, web3, blockchain, virtual reality, augmented reality, big data, IoT, and wearables, all of which were claimed as revolutionary but failed to be so.
The market dynamics that drove big data are now driving AI. Since there isn’t a real business value, the focus is on maintaining hype and media attention to keep valuations high and companies afloat.
Capabilities and Limitations of AI
At its core, AI, particularly the kind we’re excited about today, operates on complex algorithms and vast amounts of data. These models can generate human-like text, predict outcomes based on data patterns, and even assist in creating art or music. However, it’s essential to understand that these systems don’t possess true intelligence or understanding. They mimic intelligent behavior but don’t actually “think.”
Take ChatGPT, for example. It’s capable of generating coherent and contextually relevant text, but it does so based on probabilities derived from its training data. If you ask it a question with an answer outside its training data, it can stumble, sometimes spectacularly. This limitation was evident when I asked it to count the 21st letter in a sentence, and it confidently gave me the wrong answer, unable to grasp the concept of letters fully.
This brings us to a critical point: AI, as advanced as it seems, has limitations. It can’t create wholly original ideas or understand the nuances of human experiences.
It’s a tool; a very sophisticated one, but a tool nonetheless. Many of the tasks AI performs today, like proofreading text or generating code snippets, are things traditional software has been capable of for years.
The Hype Machine
Corporations have a vested interest in perpetuating AI hype. The mere mention of AI can send stock prices soaring. Investors, eager to get in on the next big thing, pour money into companies claiming to be on the cutting edge of AI technology. This phenomenon is reminiscent of the metaverse craze, where every company tried to shoehorn their products into the metaverse narrative, diluting the term to the point of meaninglessness.
Nvidia’s meteoric rise is a prime example. The company, once known for its gaming GPUs, saw its value skyrocket as it positioned itself as a leader in AI technology. Microsoft’s close relationship with OpenAI and its integration of AI into its products is another case of AI being used as a buzzword to drive investment and interest.
But this raises an important question: Are these companies genuinely advancing AI, or are they simply riding the wave of hype for financial gain? The reality is likely a mix of both. While there are genuine advancements in AI, there’s also a significant amount of marketing fluff designed to attract investors.
Real-World Applications and Misconceptions
One of the most fascinating aspects of AI is its real-world applications. From helping write computer programs to automating translations and transcriptions, AI is undeniably useful. However, much of what is branded as AI is really just advanced software that’s been around for a while. This misbranding can lead to unrealistic expectations about what AI can achieve.
Consider Amazon’s cashier-less stores. Many assumed that sophisticated AI systems managed these stores, but it turns out they likely relied on human monitors watching customers via cameras. This kind of revelation underscores the gap between what AI is believed to be capable of and what it actually does.
Another example is Google’s AI initiatives, which, in a rush to compete with ChatGPT, led to some embarrassing mistakes. The company paid millions for access to Reddit posts to train their AI, resulting in hilariously inaccurate responses.
These incidents highlight the challenges of creating AI that can reliably handle complex, real-world tasks.
The Potential and Pitfalls of AI
Despite its limitations, AI has the potential to bring significant benefits. Natural language programming could democratize coding, making it accessible to people without technical backgrounds. AI can compile and analyze vast amounts of data quickly, providing insights that would take humans much longer to uncover. Automatic translations and transcriptions make information more accessible across language barriers.
But there’s a catch. For AI to be truly transformative, it needs to be more than just good; it needs to be nearly perfect, especially in critical applications. Consider scenarios like driving a school bus or managing the finances of a large corporation. These are high-stakes tasks where mistakes can have severe consequences. Humans, despite their imperfections, offer accountability – something AI lacks.
The goal of achieving artificial general intelligence (AGI) – a form of AI that can perform any intellectual task a human can – remains a distant and uncertain prospect. AGI would require AI to learn and adapt to tasks it wasn’t explicitly trained for, a feat that current technology is far from achieving. The pursuit of AGI is akin to aiming for the stars: inspiring but fraught with challenges and uncertainties.
AI Safety and Ethical Concerns
Another layer of the AI debate involves safety and ethics. As AI becomes more integrated into our lives, the potential for misuse grows. If AI systems were to replace traditional search engines, the entities controlling these systems could manipulate information to serve their interests. This raises concerns about misinformation and the potential for AI to become a tool for controlling narratives and shaping public opinion.
The term “hallucination” is often used to describe AI errors, which is misleading. It anthropomorphizes the technology, suggesting it has human-like experiences. In reality, AI doesn’t “hallucinate”; it simply processes data and makes predictions based on that data. When it gets things wrong, it’s not due to a human-like error but a limitation in its programming or data.
Sustainability Problems with AI
One of the critical sustainability issues with AI is the scarcity of training data. Large language models (LLMs) like GPT-4 require vast amounts of human-generated text to improve their capabilities. A recent study highlighted that the available data might be exhausted within a few years. This scarcity poses a significant challenge to the continued development and enhancement of these models. As training data becomes scarce, it will be increasingly difficult to improve the AI’s performance, leading to a plateau in advancements.
Training these models is incredibly resource-intensive, both in terms of computational power and energy consumption. For instance, GPT-4 and similar models require extensive GPU clusters to process the data, leading to significant energy usage. This not only makes the development of AI expensive but also raises concerns about the environmental impact. As AI technology scales, the energy requirements will only increase, exacerbating these sustainability issues.
The economic viability of AI is another major concern.
Many AI companies, including those behind ChatGPT, are currently operating at a loss. ChatGPT, for example, reportedly loses $700,000 daily.
This economic strain raises questions about the long-term sustainability of AI businesses. If these technologies do not become profitable, it could lead to a market collapse, affecting the entire tech industry.
Limitations with Current Models
ChatGPT
ChatGPT-4 represents an iterative improvement over its predecessors. While it offers new features such as faster response times and the ability to interact with audio and video, the core intelligence remains largely the same. These enhancements are more about improving user experience than a fundamental leap in AI capability.
The future development of LLMs like ChatGPT-4 is threatened by the aforementioned scarcity of training data. As these models require exponentially more data to achieve incremental improvements, the availability of high-quality, human-generated data becomes a limiting factor. This challenge further emphasizes the need for innovative approaches to sourcing and utilizing data.
AI Video Generators
Sora, a video generator developed by ChatGPT’s creators, exemplifies the current limitations of generative AI. While it can produce video content, the quality is often poor, with frequent “hallucinations” where the AI generates implausible or incorrect visuals. For instance, attempts to create a simple video of a person walking often result in distorted or nonsensical imagery.
Generating high-quality video content with AI requires immense computational resources and time. For instance, producing a short video clip with Sora can take several hours of processing, making it impractical for many real-world applications. The energy and computational costs associated with these processes are also substantial, further questioning the technology’s sustainability.
Despite the excitement around video generators like Sora, they face significant hurdles in practical deployment. The technology is not yet capable of producing consistently high-quality, usable content. Moreover, the economic model for these tools is unclear, as the high costs and technical limitations make it challenging to justify their use over traditional video production methods.
The AI Bubble Analogy
Drawing parallels between AI and the dot-com bubble is not far-fetched. During the dot-com era, the internet was indeed transformative, but not every internet-based company was a good investment. Many failed because they couldn’t live up to the hype. Similarly, while AI has transformative potential, not every AI venture will succeed. The hype inflates expectations and can lead to a bubble that bursts when reality fails to meet those expectations.
Yet, just as the internet persisted and thrived post-bubble, AI will continue to evolve and find its place. The key is to temper expectations and focus on realistic, incremental advancements rather than expecting a sudden revolution.
The Rise of AI Skepticism
Skepticism about the actual capabilities of AI is reminiscent of the initial excitement and subsequent disillusionment experienced during the dot-com bubble. The dot-com era saw a surge of investments in internet-based companies, many of which lacked solid business models. Investors poured money into these ventures, driven by the fear of missing out. Eventually, the bubble burst, leading to significant financial losses.
Similarly, the AI hype has attracted massive investments, often overshadowing the technology’s actual capabilities. While AI has made impressive strides, the limitations and challenges are becoming increasingly apparent. For instance, Google’s Gemini AI, despite its promising demos, failed to deliver consistent results upon independent testing. This discrepancy between expectations and reality is a key factor in the growing skepticism towards AI.
The Role of Media in AI Hype
Media outlets, driven by the need for sensational stories, often focus on the most dramatic and futuristic aspects of AI. Headlines often highlight AI’s potential to revolutionize industries and change the world, creating a sense of urgency and excitement. This can mislead and create unrealistic expectations among the public and investors.
The reality is that AI, while powerful, is not a panacea. It has its limitations and challenges, It is a tool that requires careful implementation and management to be effective.
The Impact on Talent and Education
There is a growing demand for AI skills, leading to an influx of new graduates entering the field. Universities and training programs are rapidly expanding their offerings to meet this demand.
However, this rush to capitalize on the AI trend can lead to a gap between education and industry needs. Graduates may find that the skills they have acquired do not match the practical requirements of the job market. Additionally, the emphasis on AI can overshadow other important areas of study, leading to an imbalance in the talent pool.
The Potential of Open-Source AI
The open-source community has a long history of contributing to technological advancements, and AI is no exception. Open-source projects like TensorFlow and PyTorch have democratized access to powerful AI tools, enabling more people to experiment and innovate.
Open-source AI can counterbalance the dominance of big tech companies, fostering a more inclusive and collaborative approach to AI development. By supporting open-source initiatives, we can ensure that AI progresses in a way that benefits society as a whole, rather than just a select few.
The Importance of Regulation and Policy
Allowing big tech companies to self-regulate is not sufficient. These companies are driven by profit motives, which can conflict with the broader public interest.
Policymakers need to be informed and proactive in addressing the ethical and safety concerns like data privacy, algorithmic bias that are associated with AI. Relevant authorities should be fully prepared to deal with the possibility that This includes issues like data privacy, algorithmic bias, and the potential for AI t0 exacerbate social inequalities.
Overall, robust regulatory frameworks are needed to hold them accountable and ensure that AI is used responsibly.
The Need for Critical Thinking and Scrutiny
As AI continues to evolve, it is essential to maintain a critical perspective. Not every innovation will lead to a profitable business, and not every AI application will be transformative. Investors, developers, and policymakers need to scrutinize AI projects carefully, separating genuine advancements from marketing hype.
This critical approach will help ensure that AI develops in a way that is sustainable and beneficial for society. It will also help avoid the pitfalls of past tech bubbles, where unbridled enthusiasm led to significant financial and social consequences.
The Future of AI: Opportunities and Challenges
Looking ahead, the future of AI holds both opportunities and challenges. Realizing AI’s potential requires addressing the technology’s limitations and ethical concerns too.
Key areas of focus for maximum and ethical technological advancement should include:
- Transparency and Accountability: AI systems need to be transparent and accountable. This includes making the decision-making processes of AI algorithms understandable to humans and ensuring that there is accountability for the outcomes.
- Ethical AI: Developing ethical AI involves addressing issues like bias, fairness, and inclusivity. AI systems should be designed to mitigate bias and ensure that they serve all segments of society equitably.
- Collaboration and Inclusivity: The development of AI should be a collaborative effort that includes diverse perspectives. This includes engaging with stakeholders from different sectors, including academia, industry, government, and civil society.
- Education and Workforce Development: Preparing the workforce for the AI-driven future is crucial. This includes not only technical training but also fostering critical thinking and ethical considerations in AI education.
- Sustainable AI: The environmental impact of AI is an emerging concern. Developing energy-efficient AI systems and considering the environmental footprint of AI development is essential for long-term sustainability.
Personal Reflections
As I explore the world of AI, I find myself oscillating between excitement and skepticism. On one hand, the potential for AI to simplify tasks, enhance productivity, and open up new possibilities is genuinely thrilling. On the other hand, the overhyped promises and frequent misrepresentations are concerning.
I’ve seen firsthand how AI can assist in writing, providing suggestions and corrections that improve my work. But I’ve also encountered its limitations, like when it confidently provided incorrect answers or failed to understand context. These experiences highlights the importance of using AI as a tool, not a crutch.
The future of AI is undoubtedly bright, but it’s crucial to approach it with a balanced perspective.
While it’s easy to get swept up in the hype, we must remain grounded in the realities of what AI can and cannot do. The path to AGI, if it’s even possible, will be long and fraught with challenges.
In the meantime, we should focus on leveraging AI’s strengths and addressing its weaknesses.
Conclusion
Is AI a bubble? In some ways, yes. The hype surrounding AI has inflated expectations to an unsustainable level, much like the dot-com boom. However, this doesn’t mean AI is without value or potential. The technology is here to stay, and it will continue to evolve and integrate into our lives in meaningful ways.
The key is to navigate this landscape with a critical eye, separating genuine advancements from marketing fluff. Supporting open-source initiatives and robust regulations can help ensure AI development remains aligned with societal goals rather than purely corporate interests.
AI undoubtedly holds immense potential, but we must approach it with caution and a healthy dose of skepticism. By doing so and by learning from history, we can avoid the pitfalls of past tech bubbles and pave the way for a future where AI truly enhances our lives.